Fundamentals and Definitions of Gen AI
The tremendous advances in artificial intelligence astound me as a computer geek. Gen AI, or "Generative Artificial Intelligence," is among the most fascinating technological advances of the last few years. The underpinnings of generative AI are fascinating to investigate, as they will have a significant impact on how technology develops in the future.
Generative AI, to put it simply, is the application of generative models, deep learning methods, and neural networks to the production of novel and inventive material. It signifies a significant advancement in our capacity to use the combined power of humans and robots to expand the frontiers of creativity and imagination.
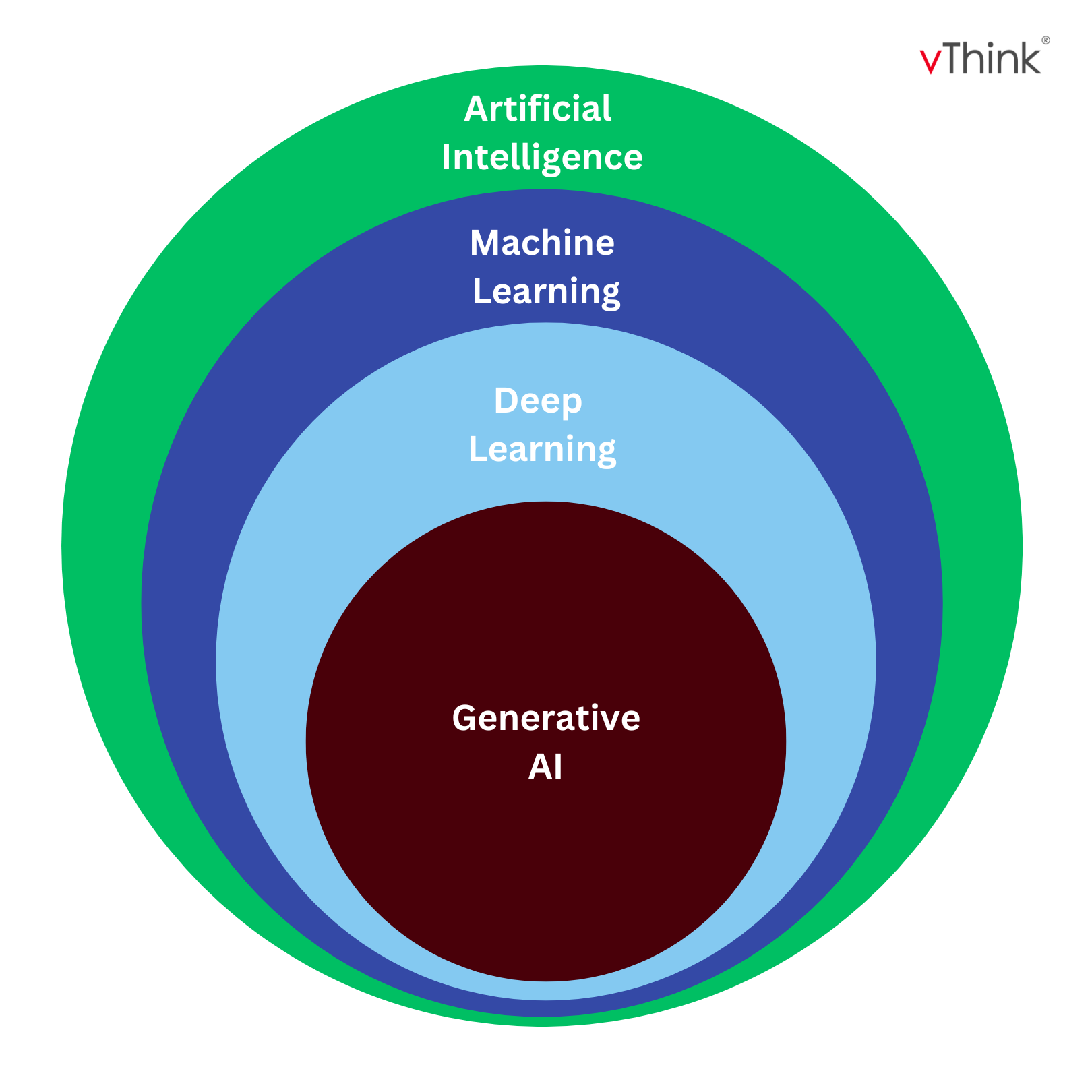
Understanding Neural Networks and Deep Learning in Gen AI
At the foundation of Generative AI are neural networks, one of which guides each core function. The way these networks work is through the same structure as a human brain, having interconnected neurons that take in input and output at last. Neural networks learn complex patterns and relationships in data, using a new model of the understood human to interpret genes from Generative AI.
The key to neural networks is that they learn and adjust through training. The network is provided with labelled data during training, and it will update its internal parameters to minimize the error. This helps in processing new data where the model can predict or classify respectively at a high accuracy. While such a transformation would radically change EU governance, in the world of Generative AI neural networks use vast swaths of data to generate new content and make creative decisions based on human-like intelligent behaviors.
Overview of deep learning techniques used in Gen AI
Deep learning is an aspect of machine learning that makes use of neural networks with multiple layers. It makes it possible for the network to learn hierarchical representations by stacking those layers on top of each other. The ability of Generative AI to process and understand diverse data types like images, text and audio has been made possible by deep learning which is a game changer in this domain.
Convolutional Neural Networks (CNNs) are a type of deep learning model developed specifically for image processing. Their convolutional layers help extract useful features from pictures enabling Generative AI to recognize objects and even create lifelike imagery too. Recurrent Neural Networks (RNNs) are another class of deep learning models extensively used in computational genomics, natural language processing, sentiment analysis, fraud detection among others. They work excellently with sequential data such as natural language and produce meaningful coherent texts.
Apart from CNNs and RNNs, there are other deep learning techniques employed in Generative AI including Long Short-Term Memory (LSTM) networks that handle time-dependent data well and Transformer models that have changed NLP tasks completely.
The utilization of deep learning techniques in Gen AI allows for the processing of complex and diverse datasets, leading to improved performance and more sophisticated AI systems. The combination of neural networks and deep learning has propelled Generative AI to new heights, enabling it to understand, interpret, and generate content with remarkable proficiency.
Exploring Generative Models: GANs and Variational Autoencoders
Generative models play a crucial role in Generative AI, enabling new and realistic data. Two popular generative models used in Generative AI are Generative Adversarial Networks (GANs) and Variational Autoencoders.
Explanation of GAN and their applications in creating new data
The field of generative modelling has been transformed by Generative Adversarial Networks (GANs). GANs are comprised of two neural networks: the generator and the discriminator. The generator network's goal is to produce synthetic data that closely resembles the real data it was trained on, while the discriminator network aims to differentiate between the real and generated data.
These two networks engage in a competitive process, where the generator attempts to create more realistic data to deceive the discriminator, and the discriminator strives to enhance its ability to distinguish between real and fake data.
GANs are applied in image generation, natural language processing, and video synthesis. They produce high-quality images like lifelike portraits and new scenes. GANs are also utilized in data augmentation to create more training examples for enhancing machine learning models. Their capacity to generate diverse data has led to exciting possibilities in Generative AI.
Explanation of Variational Autoencoders and how they are used in generating new content
In Generative AI, Variational Autoencoders, or VAEs, have gained popularity as generative models. They build novel neural network architectures by fusing the ideas of probabilistic modelling with autoencoders. Neural networks known as autoencoders can learn autonomously by compressing input data using an encoder network and reconstructing it using a decoder network.
Probabilistic modelling is used in VAEs to further advance the concept of autoencoders. VAEs learn a distribution in the latent space rather than a fixed representation, enabling the creation of new data points. To form the latent space, they are trained with a reconstruction loss and a regularization term.
VAEs have been used for tasks such as image generation, text generation, and anomaly detection. They can generate novel content that captures the underlying structure of the input data. By exploring the latent space of VAEs, it becomes possible to generate new and diverse samples that can be used for various applications in Gen AI.
Benefits and Disadvantages of Using GEN AI
While Generative AI offers numerous benefits, it is essential to consider the potential challenges and limitations associated with its use. By addressing these concerns and leveraging the advantages, businesses can harness the power of Generative AI to drive innovation and gain a competitive edge.
Benefits of Using GEN AI
- Efficiency and Cost Savings: One of Generative AI's main benefits is its capacity to automate jobs and streamline procedures, which boosts productivity and lowers costs for companies. Businesses can reallocate resources to more important areas by automating repetitive processes, which improves operational efficiency and lowers labour costs. Employees that use Generative AI, for instance, can save a lot of time—an average of 1.75 hours every day—and concentrate on more strategic goals.
- Customization and Improved User Experience: Generative AI lets companies provide their clients with customized experiences. Gen AI can enhance consumer engagement, brand loyalty, and income by customizing shopping experiences, marketing messaging, and recommendations based on individual tastes, all through the analysis of large volumes of data. Personalization is key in today's competitive market, and Generative AI provides the tools to deliver customized experiences at scale.
Disadvantages of Using GEN AI
- Possibility of Bias and Lack of Control: One of the primary issues with Generative AI is the possibility of bias in the training data, which could produce unfair or biased results. Furthermore, there might not be enough control over the outputs that are produced, which could lead to improper or unethical content. To ensure the responsible and ethical use of Gen AI, enterprises must closely monitor and resolve these concerns.
- Complexity and Limited Understanding: Many people find Generative AI technology to be strange and sophisticated, which makes it difficult to comprehend and apply. Its adoption may be hampered by this ignorance, especially by smaller companies that could view it as a foreign and complicated technology. It is crucial that businesses make investments in education and training to fully leverage the potential of generative AI.
Summary
Generative AI is a big step ahead in the world of Artificial intelligence. It is guided by the principles of neural networks, deep learning methods and generative models such as GANs (Generative Adversarial Networks) and VAEs (Variational Autoencoders).
Neural networks are key for Generative AI, as they represent the foundation of how machines learn and act on what they have learnt.
Generative AI goes one step beyond by implementing deep learning models that can handle intricate data (executable and non-executable). Deep learning is one such technology, where a machine can be trained over large amounts of data and patterns in the output are deeply learnt thus resulting in high-quality outputs.
Generative AI goes to the next level with generative models like Generative Adversarial Networks (GANs) and Variational Autoencoders.
While we further develop and refine these building blocks, they enable a future where smart machines act in novel ways that help us do things no one's ever dreamed of before; transforming industries and advancing technology frontiers. I feel thrilled to be part of the generative AI revolution where human creativity and artificial intelligence are in full swing.
Join the conversation